Mathématiques pour les IFS, fractales & jeu du chaos
Cadre mathématique et démonstrations
Distances
Une distance est une application

-
(symétrie)
-
(séparation)
- Pour tous
,
et
,
(inégalité triangulaire)
Par exemple, en géométrie dans le plan




-
-
- pour tout point
,
Si le plan est rapporté à un repère orthonormé, et que


![\[d(A,B)=AB=\sqrt{\left( x-x'\rp^2+\left( y-y'\rp^2}\]](fich-chaos-IMG/193.png)
est une distance, la distance euclidienne.
On peut définir de nombreuses distances, suivant le contexte, les éléments dont on cherche à mesurer la distance: distances entre des points, entre des fonctions, entre des matrices, … et aussi, ce qui nous intéresse plus ici, entre des ensembles géométriques de points (c'est-à-dire des figures géométriques, ou encore des images).
Distance et norme
Un espace normé est un espace muni d'une norme, un espace métrique est lui muni d'une distance.Une norme, notée en général


La réciproque n'est par contre pas toujours vraie: une distance peut ne pas découler d'une norme.
En d'autres termes, un espace normé est nécessairement aussi métrisé (ou métrique), par contre un espace métrique n'est pas toujours normé.
La notion de norme est plus fine que celle de distance.
Une distance entre éléments d'un espace permet par contre aussi d'induire un distance entre des ensembles d'éléments: c'est la distance de Hausdorff.
Distance de Hausdorff
Définition et propriété
En traitement d'image par exemple, on cherche à donner un sens à la proximité de deux images entre elles: deux images, par exemple une floue et la même filtrée et plus nette, sont elles "proches" entre elles.
Plus précisément sur une série d'images filtrées et traîtées, laquelle est la plus "proche" de l'image souhaitée.
Comment quantifier cette "proximité" ? Comment vérifier que deux motifs, ou parties d'images sont identiques ?
Une distance est un outil mathématique qui permet exactement de définir la notion de proximité.
La distance de Hausdorff est une distance qui permet de mesurer la différence entre des ensembles, de points par exemple (c'est à dire des images).
Pour définir cette distance de Hausdorff, on a besoin de la définition de la distance d'un point à un ensemble, puis de celle de voisinage d'un ensemble.
Définition: distance d'un point à un ensemble
La distance d'un point
à un ensemble
est la plus petite des
distances entre
et les points de
:




![\[d(x,A)=\inf\Bigl\{ d(x,y) , y\in A \Bigr\}\]](fich-chaos-IMG/200.png)
(3,1)
\rput(1.1,2){\Large$A$}
\rput(0,5){\large$\tm$}\rput(-.25,5.2){\large$x$}
\psline[linewidth=1.5pt,linecolor=magenta,arrowsize=8.5pt]{<->}(0,5)(0.4,2.82)
\rput(1.1,4){\large\magenta$d(x,A)$}
\end{pspicture}\]](fich-chaos-IMG/201.png)
Définition: Voisinage
On appelle voisinage, ou
d'un ensemble
de points du plan
l'ensemble des points


![\[V_r(A)=\Bigl\{ x \text{ tel que } d(x,A)<r \Bigr\}\]](fich-chaos-IMG/204.png)
(3,1)
\rput(1.1,2){\Large$A$}
\psellipse[fillstyle=vlines,hatchsep=2.1em,hatchcolor=blue](2,2)(4,1.9)
\psellipse[linestyle=none,fillstyle=solid,fillcolor=white](.42,3.15)(.29,.28)
\psellipse[linestyle=none,fillstyle=solid,fillcolor=white](.42,3.15)(.29,.28)
\psellipse[linestyle=none,fillstyle=solid,fillcolor=white](4,3.15)(.5,.28)
\psline[linewidth=2pt,linecolor=magenta,arrowsize=7pt]{<->}(4,2.7)(4.5,3.5)
\rput(4,3.2){\large\magenta$r$}
\psellipse[linestyle=none,fillstyle=solid,fillcolor=white](.42,3.15)(.29,.28)
\rput(.2,3.1){\large\blue$V_r(A)$}
\end{pspicture}\]](fich-chaos-IMG/205.png)
Définition: distance de Hausdorff
La distance de Hausdorff entre deux ensembles
et
est
définie par
![\[d_H(A,B)=\inf\Bigl\{ r>0 \text{ tel que }
A\subset V_r(B) \text{ et } B\subset V_r(A) \Bigr\}
\]](fich-chaos-IMG/208.png)


![\[d_H(A,B)=\inf\Bigl\{ r>0 \text{ tel que }
A\subset V_r(B) \text{ et } B\subset V_r(A) \Bigr\}
\]](fich-chaos-IMG/208.png)
La distance de Hausdorff est définie à partir de la notion de voisinage, qui elle-même est dépend de la distance dans l'espace considéré.
La distance de Hausdorff n'est pas une distance sur tous les ensembles; par exemple, pour des ensembles de points non-bornés, la distance de Hausdorff n'est pas définie (ou vaut dans ce cas l'infini).
Par contre, en se limitant aux ensembles compacts, qui sont dans le plan les ensembles fermés et bornés, la distance de Hausdorff définie bien une distance: en notant


Propriété: La distance de Hausdorff est une distance
sur
.

Démonstration.
Il faut démontrer les trois propriétés d'une distance.
- Symétrie: elle est évidente, la définition de la distance étant
elle même symétrique:
.
- Séparation: Supposons
et
sont deux ensembles compacts tels que
.
Cela signifie que pour tout, on a
(et de même
).
Prenons par exemple, tel que
.
Alors, il existetel que
.
Comme pour tous entierset
on a d'après l'inégalité triangulaire
, la suite
est de Cauchy. Comme on est dans un espace complet, cette suite converge, ce qui signifie qu'il existe
tel que
.
Enfin, commeest compact, et que pour tout
,
, on a aussi que
.
Ainsi, en passant à la limite, on obtientsoit donc
.
Finalement, on a donc.
En raisonnant de même en interchangeantet
, on obtient aussi
, et donc
.
- Inégalité triangulaire:
soit
,
et
trois ensembles compacts, on cherche à montrer que,
.
On note,
et
.
On a alorset
.
Ainsi,
Ord'après le lemme suivant.
Ainsi,.
De même, on arrive symétriquement à.
On a ainsi,.
Lemme:
Pour tout ensemble
, et
et
,
on a
.




Démonstration.
Soit
,
alors il existe
tel que
.
De même, comme
, il existe
tel que
.
D'après l'inégalité triangulaire, on a
,
ce qui signifie que
.


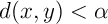
De même, comme



D'après l'inégalité triangulaire, on a


Exemples
Exemple 1:
On cherche par exemple la distance de Hausdorff entre les ensembles




(2,2)(-2,2)(-2,-2)
\pscircle[linecolor=red,fillstyle=hlines,hatchcolor=red,hatchsep=1.1em](1,.5){2}
\pscircle[fillstyle=solid,fillcolor=white,linestyle=none](-1.7,-1.6){.25}
\rput(-1.7,-1.5){\large\blue$A$}
\rput(2.5,.3){\large\red$B$}
\end{pspicture}\]](fich-chaos-IMG/270.png)
On trace le plus petit voisinage de




(2,2)(-2,2)(-2,-2)
\pscircle[fillstyle=solid,fillcolor=white,linestyle=none](-1.7,-1.6){.25}
\rput(-1.7,-1.5){\large\blue$A$}
\pscircle[linecolor=red,fillstyle=hlines,hatchcolor=red,hatchsep=1.1em](1,.5){2}
\pscircle[fillstyle=solid,fillcolor=white,,linestyle=none](2.5,.3){.3}
\rput(2.5,.3){\large\red$B$}
\psline[arrowsize=6pt,linecolor=blue,linewidth=1.5pt]{<->}(-3,1)(-2,1)
\rput(-2.5,1.3){\large\blue$r_1$}
\psline(3,-2)(3,2)
\psline(2,3)(-2,3)
\psline(-3,2)(-3,-2)
\psline(-2,-3)(2,-3)
\psarc(2,2){1}{0}{90}
\psarc(-2,2){1}{90}{180}
\psarc(-2,-2){1}{180}{270}
\psarc(2,-2){1}{270}{360}
\end{pspicture}\]](fich-chaos-IMG/275.png)
(12,2)(8,2)(8,-2)
\pscircle[linecolor=red,fillstyle=hlines,hatchcolor=red,hatchsep=1.1em](11,.5){2}
\psline[arrowsize=6pt,linecolor=red,linewidth=1.5pt]{<->}(13,.5)(14.95,.5)
\rput(14,.8){\large\red$r_2$}
\pscircle(11,.5){3.95}
\pscircle[fillstyle=solid,fillcolor=white,linestyle=none](8.3,-1.6){.25}
\rput(8.3,-1.5){\large\blue$A$}
\pscircle[fillstyle=solid,fillcolor=white,,linestyle=none](12.5,.3){.3}
\rput(12.5,.3){\large\red$B$}
\end{pspicture}\]](fich-chaos-IMG/276.png)
On a ici

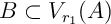
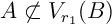
Par contre
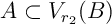

Ainsi,

Exemple 2: La distance de Hausdorff est une distance sur



Par exemple, si

![$A=]0;1[\tm]0;1[$](fich-chaos-IMG/287.png)

![$\overline{A}=[0;1]\times[0;1]$](fich-chaos-IMG/289.png)
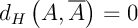

Application contractante
Une application contractante est une application qui rapproche les points, c'est-à-dire que les images de deux points sont plus proches que les points eux-même. Plus précisément,
Définition: application contractante
Une application
est contractante
si il existe un nombre réel
tel que, pour tous
et
,




![\[d\left( f(x),f(y)\rp\leqslant k\,d\left( x,y\rp\]](fich-chaos-IMG/296.png)
Il faut ici faire attention à la distance utilisée. Ce n'est pas forcément la même distance à gauche et à droite de l'inégalité précédente. La distance euclidienne peut être utilisée s'il s'agit de distance entre points du plan, celle de Hausdorff pour des ensembles de points.
L'opérateur de Hutchinson
est contractant
On peut maintenant revenir sur l'opérateur de Hutchinson.
Propriété: L'opérateur de Hutchinson
est contractant
sur
pour la distance de Hausdorff.


Pour démontrer cette propriété, qui est la propriété fondamentale garantissant l'existence et l'unicité de l'attracteur d'un IFS, on a besoin de résultats intermédiaires.
Lemme 1:
Pour tous ensemble
et
, et réel
,
on a
.




Démonstration.
Soit
.
et
jouant des rôles symétriques, on peut supposer
.
Il existe donc
tel que
.
Mais alors, on a aussi
et toujours
,
ce qui signifie que
.




Il existe donc


Mais alors, on a aussi

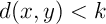

Lemme 2:
Soit
une contraction du plan de rapport
,
alors, pour tous ensembles
et
,
![\[d_H\left( f(A),f(B)\rp\leqslant d_H(A,B)\]](fich-chaos-IMG/317.png)




![\[d_H\left( f(A),f(B)\rp\leqslant d_H(A,B)\]](fich-chaos-IMG/317.png)
Démonstration.
On pose
.
Soit
, alors il existe
tel que
.
Comme
, on a
,
et donc il existe
tel que
.
On pose alors
et on a, comme
est
-contractante,
![\[
d\left( a',b'\rp=d\left( f(a),f(b)\rp
\leqslant r d(a,b)
\leqslant rk\]](fich-chaos-IMG/329.png)
En d'autres termes, on a trouvé que pour tout
,
il existe
tel que
,
d'où
.
De même, en inversant les rôles de
et
,
on aboutit à
.
On a donc finalement,
.

Soit



Comme



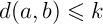



![\[
d\left( a',b'\rp=d\left( f(a),f(b)\rp
\leqslant r d(a,b)
\leqslant rk\]](fich-chaos-IMG/329.png)
En d'autres termes, on a trouvé que pour tout




De même, en inversant les rôles de



On a donc finalement,

Lemme 3:
Pour tous compacts
et
du plan,
![\[d_H\left( A\cup B,C\cup D\rp\leqslant \max\Bigl\{d_H(A,C),d_H(B,D)\Bigr\}\]](fich-chaos-IMG/340.png)


![\[d_H\left( A\cup B,C\cup D\rp\leqslant \max\Bigl\{d_H(A,C),d_H(B,D)\Bigr\}\]](fich-chaos-IMG/340.png)
Démonstration.
On pose
et
.
On a alors, par définition de la distance de Hausdorff,
![\[A\subset V_d(C) \ \text{ et } \ C\subset V_d(A)\]](fich-chaos-IMG/343.png)
et de même
![\[B\subset V_{d'}(D) \ \text{ et } \ D\subset V_{d'}(B)\]](fich-chaos-IMG/344.png)
et donc, en notant
,
![\[A\subset V_k(C) \ \text{ et } \ C\subset V_k(A)\]](fich-chaos-IMG/346.png)
et de même
![\[B\subset V_k(D) \ \text{ et } \ D\subset V_k(B)\]](fich-chaos-IMG/347.png)
Or, d'après le lemme 1,
et donc,
![\[A\cup B\subset V_k(C)\cup V_k(D) \subset V_k\left( C\cup D\rp\]](fich-chaos-IMG/349.png)
et de même
![\[C\cup D\subset V_k(A)\cup V_k(B) \subset V_k\left( A\cup B\rp\]](fich-chaos-IMG/350.png)
et on a donc,
![\[d_H\left( A\cup B,C\cup D\rp\leqslant k=\max\left\{}\newcommand{\ra}{\right\} d,d'\ra
=\max\Bigl\{d_H(A,C),d_H(B,D)\Bigr\}\]](fich-chaos-IMG/351.png)


On a alors, par définition de la distance de Hausdorff,
![\[A\subset V_d(C) \ \text{ et } \ C\subset V_d(A)\]](fich-chaos-IMG/343.png)
et de même
![\[B\subset V_{d'}(D) \ \text{ et } \ D\subset V_{d'}(B)\]](fich-chaos-IMG/344.png)
et donc, en notant

![\[A\subset V_k(C) \ \text{ et } \ C\subset V_k(A)\]](fich-chaos-IMG/346.png)
et de même
![\[B\subset V_k(D) \ \text{ et } \ D\subset V_k(B)\]](fich-chaos-IMG/347.png)
Or, d'après le lemme 1,

et donc,
![\[A\cup B\subset V_k(C)\cup V_k(D) \subset V_k\left( C\cup D\rp\]](fich-chaos-IMG/349.png)
et de même
![\[C\cup D\subset V_k(A)\cup V_k(B) \subset V_k\left( A\cup B\rp\]](fich-chaos-IMG/350.png)
et on a donc,
![\[d_H\left( A\cup B,C\cup D\rp\leqslant k=\max\left\{}\newcommand{\ra}{\right\} d,d'\ra
=\max\Bigl\{d_H(A,C),d_H(B,D)\Bigr\}\]](fich-chaos-IMG/351.png)
On peut maintenant démontrer la propriété fondamentale sur l'opérateur de Hutchinson.
Démonstration.
Soit
et
deux ensembles compacts du plan, alors
![\[d_H\left( F(A),F(B)\right)
=d_H\Bigl( f_1(A)\cup f_2(A)\cup\dots\cup f_N(A),
f_1(B)\cup f_2(B)\cup\dots\cup f_N(B)\Bigr)
\]](fich-chaos-IMG/354.png)
soit, en utilisant le lemme 3,
![\[\begin{array}{ll}
d_H\left( F(A),F(B)\right)
&=d_H\Bigl( f_1(A)\cup D,
f_1(B)\cup E\Bigr)\\[1em]
&\leqslant\max\Bigl\{ d_H\Bigl( f_1(A),f_1(B)\Bigr) ,
d_H\left( D,E\right) \Bigr\}
\enar\]](fich-chaos-IMG/355.png)
avec
et
.
Or, comme
est
-contractante, d'après le lemme 2,
,
d'où
![\[d_H\left( F(A),F(B)\right)
\leqslant \max\Bigl\{ r_1d_H (A,B), d_H(D,E)\Bigr\}\]](fich-chaos-IMG/361.png)
On continue avec les ensemble
et
:
![\[\begin{array}{ll}
d_H(D,E)
&=d_H\Bigl( f_2(A)\cup f_3(A)\cup\dots\cup f_N(A),
f_2(B)\cup f_3(B)\cup\dots\cup f_N(B)\Bigr) \\[1em]
&=d_H\Bigl( f_2(A)\cup D',f_2(B)\cup E'\Bigr)
\enar\]](fich-chaos-IMG/364.png)
avec
et
d'où, à nouveau à l'aide du lemme 3,
![\[\begin{array}{ll}
d_H(D,E)\leqslant
\max\Bigl\{ d_H\Bigl(f_2(A),f_2(B)\Bigr),d_H\left( D',E'\rp\Bigr\}
\enar\]](fich-chaos-IMG/367.png)
avec, d'après le lemme 2,
.
En poursuivant ainsi, on arrive donc enfin à:
![\[\begin{array}{ll}
d_H\left( F(A),F(B)\rp&\leqslant
\max\Bigl\{ r_1d_H(A,B),r_2d_H(A,B),\dots,r_Nd_H(A,B)\Bigr\}\\[1.2em]
&=\max\Bigl\{ r_1,r_2,\dots,r_N\Bigr\} d_H(A,B)\\[1em]
&=rd_H(A,B)
\enar\]](fich-chaos-IMG/369.png)
avec
.


![\[d_H\left( F(A),F(B)\right)
=d_H\Bigl( f_1(A)\cup f_2(A)\cup\dots\cup f_N(A),
f_1(B)\cup f_2(B)\cup\dots\cup f_N(B)\Bigr)
\]](fich-chaos-IMG/354.png)
soit, en utilisant le lemme 3,
![\[\begin{array}{ll}
d_H\left( F(A),F(B)\right)
&=d_H\Bigl( f_1(A)\cup D,
f_1(B)\cup E\Bigr)\\[1em]
&\leqslant\max\Bigl\{ d_H\Bigl( f_1(A),f_1(B)\Bigr) ,
d_H\left( D,E\right) \Bigr\}
\enar\]](fich-chaos-IMG/355.png)
avec


Or, comme



![\[d_H\left( F(A),F(B)\right)
\leqslant \max\Bigl\{ r_1d_H (A,B), d_H(D,E)\Bigr\}\]](fich-chaos-IMG/361.png)
On continue avec les ensemble


![\[\begin{array}{ll}
d_H(D,E)
&=d_H\Bigl( f_2(A)\cup f_3(A)\cup\dots\cup f_N(A),
f_2(B)\cup f_3(B)\cup\dots\cup f_N(B)\Bigr) \\[1em]
&=d_H\Bigl( f_2(A)\cup D',f_2(B)\cup E'\Bigr)
\enar\]](fich-chaos-IMG/364.png)
avec


![\[\begin{array}{ll}
d_H(D,E)\leqslant
\max\Bigl\{ d_H\Bigl(f_2(A),f_2(B)\Bigr),d_H\left( D',E'\rp\Bigr\}
\enar\]](fich-chaos-IMG/367.png)
avec, d'après le lemme 2,

En poursuivant ainsi, on arrive donc enfin à:
![\[\begin{array}{ll}
d_H\left( F(A),F(B)\rp&\leqslant
\max\Bigl\{ r_1d_H(A,B),r_2d_H(A,B),\dots,r_Nd_H(A,B)\Bigr\}\\[1.2em]
&=\max\Bigl\{ r_1,r_2,\dots,r_N\Bigr\} d_H(A,B)\\[1em]
&=rd_H(A,B)
\enar\]](fich-chaos-IMG/369.png)
avec

Théorème du point fixe
Il existe de nombreux théorèmes du point fixe en analyse. Ils permettent de démontrer l'existence et l'unicité d'une solution à des problèmes, en fournissant de plus une méthode constructive pour les déterminer (ou du moins en donner une approximation, avec une précsion souhaitée).
Le théorème du point fixe utilisé ici est celui de Banach, ou de Picard, et se place dans un espace métrique complet.
Un espace métrique est un espace dans lequel on peut mesurer, c'est-à-dire muni d'une distance telle que vue précédemment. On note justement

Théorème du point fixe de Banach / Picard:
Une application
contractante dans un espace métrique complet
admet un unique point fixe, c'est-à-dire un unique
tel que
.
De plus,
est la limite de toute suite
définie
par récurrence selon
.
On a de plus alors les majorations
![\[d\left( x_n,x\right) \leqslant k^n d\left( x_0,x\right)\]](fich-chaos-IMG/378.png)
et
![\[d\left( x_n,x\right) \leqslant \dfrac{k^n}{1-k} d\left( x_0,x_1\right)\]](fich-chaos-IMG/379.png)
où
est le rapport de contraction de
.



De plus,



On a de plus alors les majorations
![\[d\left( x_n,x\right) \leqslant k^n d\left( x_0,x\right)\]](fich-chaos-IMG/378.png)
et
![\[d\left( x_n,x\right) \leqslant \dfrac{k^n}{1-k} d\left( x_0,x_1\right)\]](fich-chaos-IMG/379.png)
où


Démonstration:
Ce théorème donne deux résultats:
l'existence et l'unicité du point fixe
.
On démontre les deux séparemment.

On démontre les deux séparemment.
- Unicité. Supposons au contraire qu'il y ait deux points fixes
et
:
et
avec
.
On aurait alorsor, comme
est contractance de rapport
, on a aussi
.
On devrait donc avoirce qui est impossible.
Ainsi, si un point fixe existe, il est nécessairement unique.
- Existence.
L'existence se démontre par le procédé construcif même utilisé tout au long de ces pages: soitune suite définie par récurrence par
.
Alors on, our tout entieret
avec, comme,
.
Ceci montre que la suiteest une suite de Cauchy, et alors, comme on est dans un espace complet, la suite converge donc vers une limite
.
Par unicité du point fixe, on voit donc que ce procédé constructif ne dépend pas du point initialde la suite: pour tout
la suite
ainsi définie converge, vers le point fixe de
.